Pain in the Brain
Pain affects the life of large amount of people. Almost everybody has had a period in their life in which they suffered from a lot of pain, by injuries, illnesses, surgeries or just spontaneously. Pain is not only a major physical and psychological burden for patients, but also has big economic and social impact on society. In Europe, it was found that over the range of one month 20.2% of all people suffer a form of pain. There is a big group among those people that experiences chronic pain which was shown to have a prevalence of up to 30%. Forty percent of those people indicate to receive inadequate treatment for their pain [1]. Even though it is already bad to have pain due to an injury, chronic pain is completely different in the sense that it can occur without a reason and keeps coming back. People with chronic pain often receive an inadequate treatment because it is very difficult to determine the reason of the chronic pain and which treatment could help to ease the pain. Therefore, there is a need for improved and new diagnostics of pain. To do so, we need to discover why, where and how our pain system is generating this spontaneous pain.
You might wonder what the connection is between pain and electrical engineering. Even though mental pain is one of the side-effects of studying electrical engineering, this is not the reason for writing this article. Diagnosing pain is very complicated and we need engineers to develop smart tools to make smart measurements. Challenges in our field include the development of specialized stimulation electrodes, medical-grade stimulation devices and software, recording signals of just a few micro-volts and analyzing large amounts of recorded data.
Moreover, it can be very helpful to look at the nervous system from an engineering point-of-view. Our body includes an incredibly complex system of nerves. The connections between those nerves comprise relatively simple circuits inside our tissue and spinal cord, and incomprehensibly large networks inside our brains. The combination of specialized stimulators and electrodes with mathematical techniques from statistics, signal processing and telecommunications engineering help to shed light on the properties of those networks.
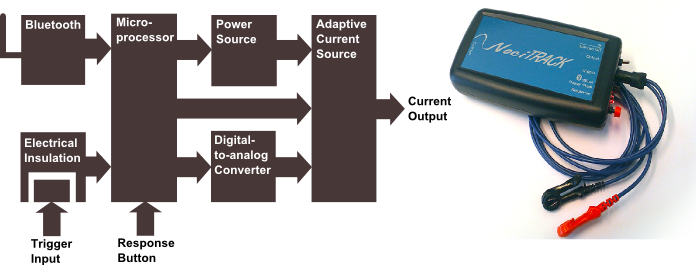
Figure 1
Stimulation
One way to measure properties of the pain system is by determining input-output relations. To provide the pain system with an input, we use small localized electrical currents to elicit action potentials in pain-specific nerves. A major development project within our group was the NociTrack AmbuStim stimulator, which is designed to provide accurate electrical stimuli to the nervous system. Figure 1 shows a block diagram with the components of this stimulator. The stimulator has to be isolated from the power network for patient safety. Therefore, we have made a stimulator which can be used wireless in most of the cases and a trigger input which is insulated from the stimulation circuit. Furthermore, it can generate arbitrary sequences of stimuli which can be programmed and adapted in real-time via LabView, providing us with an ideal interface for adaptive testing of input-output relations. In addition, this stimulator can accurately stimulate at intensities as low as 8 uA, which allows for very specific stimulation of the neurons located most nearby the electrode. The development of the AmbuStim is still ongoing, as new features are required to improve pain diagnostics.
Even though we can accurately regulate the current pulses, we cannot simply stimulate a single input. Instead, it’s input consists of billions of nerve fibers of various kinds spread out over our bodies. Generally, the fibers that provide input into the pain system consist of 3 categories (Figure 2). The largest fibers (A-beta) are responsible for the conduction of tactile signals from the skin. Even though these fibers are not responsible for the sensation of pain, they partially connect to the same pathways in the spinal cord as the pain-specific fibers. Those fibers have a smaller diameter and lie more superficially in the skin, the A-delta and C-fibers. To reduce activity of tactile neurons, which would distort the activity of the pain-specific neurons, it is necessary to stimulate the A-delta and C fibers as specific as possible.
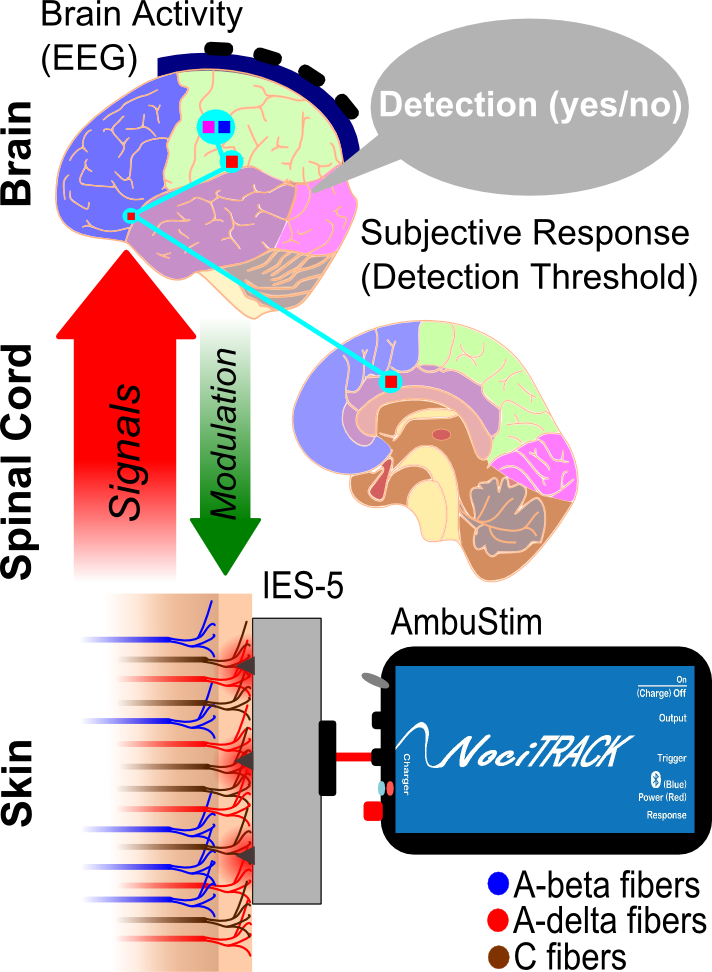
Figure 2
Another practical engineering challenge is to develop electrodes for the specific stimulation ofthese nerve fibers. Development of those electrodes involves 1) choosing the structure and materials of the electrode, 2) modeling the current density during stimulation and the associated activation of nerve fibers and 3) testing and validating the sensations those electrodes generate. One of the electrodes we developed for specific stimulation of A-delta fibers (the IES-5, Figure 2) uses micro-needles to penetrate the skin approximately 0.2 mm to come close to the A-delta and C fibers [2]. Due to the shape of the needles, the current density will only be strong enough for stimulation of nerves around the needle, excluding the more deeply located A-beta fibers. Since an electrode with needles requires sterilization, development of a needle-less electrode that is as accurate and specific as the IES-5 is a current subject of research.
Recording
It would be impossible to characterize properties of the system, if we cannot measure the output. There is a wide variety of psychophysical and physiological techniques available to measure a subject’s response to a stimulus. Because we focus on the development of clinically applicable diagnostic methods for chronic pain, the method should be quick and relatively low-cost. Until recently, we have only used psychophysical measurements to quantify the output. The field of psychophysics studies the properties of stimulus perception. Our mind is a very advanced classification system and can perceive a wide variety of stimuli: it can distinguish around a million colors, subtle tactile sensations and very low electrical currents in the skin (as small as 0.05 mA), which we use in our research. In the case of pain, the classification of your mind will differ with respect to the input it receives from the spinal cord. Therefore, by measuring the probability of your decision with respect to the stimuli, we can also observe changes in the processing of your spinal cord. One of the methods we developed used a sequence of randomized stimuli to track changes of the subject’s detection threshold over time. The detection threshold is the stimulus intensity at which 50% of the stimuli is detected, and is very sensitive to changes in the pain system, such as learning, medical interventions and external inputs to the system, such as from tactile or thermally sensitive neurons [3, 4] (Figure 3). The clinical applications of this technique are currently further researched and developed in the hospital in cooperation with anesthesiologists.
Figure 3
Even though tracking of the detection threshold has proven to be very insightful in studying several processes within the pain system, we are still looking for ways to obtain more specific and objective measures of pain. One way to acquire objective measures of sensation is by measuring the brain activity associated with stimuli. Due to enormous advances in brain research, which are mainly attributable to engineering, various techniques (PET, fMRI, MEG, EEG) are available to have a look inside the black box which is our brain. To observe electrophysiological activity with a high temporal accuracy we can use magnetoencephalography (MEG) and electroencephalography (EEG). While MEG is temporally accurate and provides a higher spatial resolution than EEG, it is only sensitive to activity of tangentially oriented neurons, while pain elicits activity in both radially and tangentially oriented neurons. Therefore, we use EEG to assess brain activity with respect to pain, which provides a good trade-off between temporal and spatial accuracy besides being relatively low-cost and easy-to-use [5]. By analysis brain activity with respect to stimulation, we can measure one step earlier in the system: the neural activity that advances stimulus detection.
Analysis of Brain Signals
In an EEG recording, we attempt to measure the activity of a small subgroup of neurons with respect to a stimulus from the surface of the scalp. As you can imagine, the signal will be very small, and the amount of noise and unrelated background activity very large! Therefore, the EEG signal offers very specific challenges in terms of processing, analysis and interpretation. Using signal processing techniques from electrical engineering and mathematics, we can filter relevant signals from this bulk of activity.
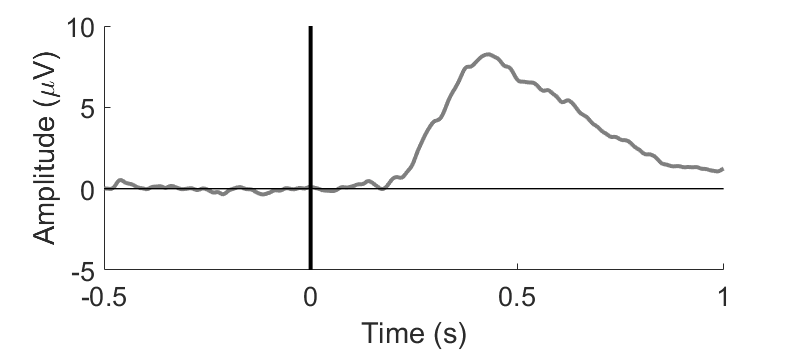
Figure 4
To understand the difficulties associated with analysis of the EEG signal, we should start at the source-level. The outer layer of the brain, the cortex, consists of many billions of neurons, each creating a small dipole field when they fire. When millions similarly oriented neurons fire synchronously, they might generate a local potential that is sufficiently high to be measured by EEG. The shape of the potential field created by those neurons will depend on their orientation. However, activity of a neuron is not necessarily related to an event: local groups of neurons can be connected to one or multiple neural networks within the brain, which communicate with other networks by generating an oscillating potential field. This means that, no matter how much you constrain your experimental conditions, there will always be a tremendous amount of background activity.
Signals related to the stimulus consist of a transient change in the potential measured by EEG (Figure 4) as well as modulations in the frequency domain. Transient changes in the potential typically have an SNR of 0.01 to 0.3, depending on the electrode position, and frequency modulations even lower. We can estimate the true signal by averaging a large amount of the same signals. This can be done by applying the same stimulus a large amount of times and measuring the associated EEG activity. If the measurement includes a range of continuous variables, the averaging is done over bins of those variables. One can imagine that, if the number of samples in a bin is low, the fit will be of poor quality: the power of the background activity scales with 1/n with respect to the number of samples.
We are currently working on the development of more sophisticated analysis methods. In our measurements, a large variety of stimulus types and amplitudes is used, which leads to exactly the aforementioned problem: averaging does not lead to an accurate estimate of the transient signal (Figure 5A) or frequency spectra. Current work has shown that we can exploit the approximately linear relation between stimulus intensity and brain activity to do a regression over all trials instead of averaging over small subsets. Where averaging minimizes the residual (η_i (τ)) in Equation 1, linear regression minimizes of the residual in Equation 2. Regression is implemented by including relevant factors as regressors (x_n). This requires the assumption that the variations with respect to the regression parameters are linear, which can be largely verified by checking model residuals. Figure 5B shows that this approach generates significantly cleaner data which can be used to study the modulation of brain signals with respect to stimulus properties. For example, this technique will be used to observe the difference between patients with chronic regional pain syndrome (CRPS) and a healthy control group.
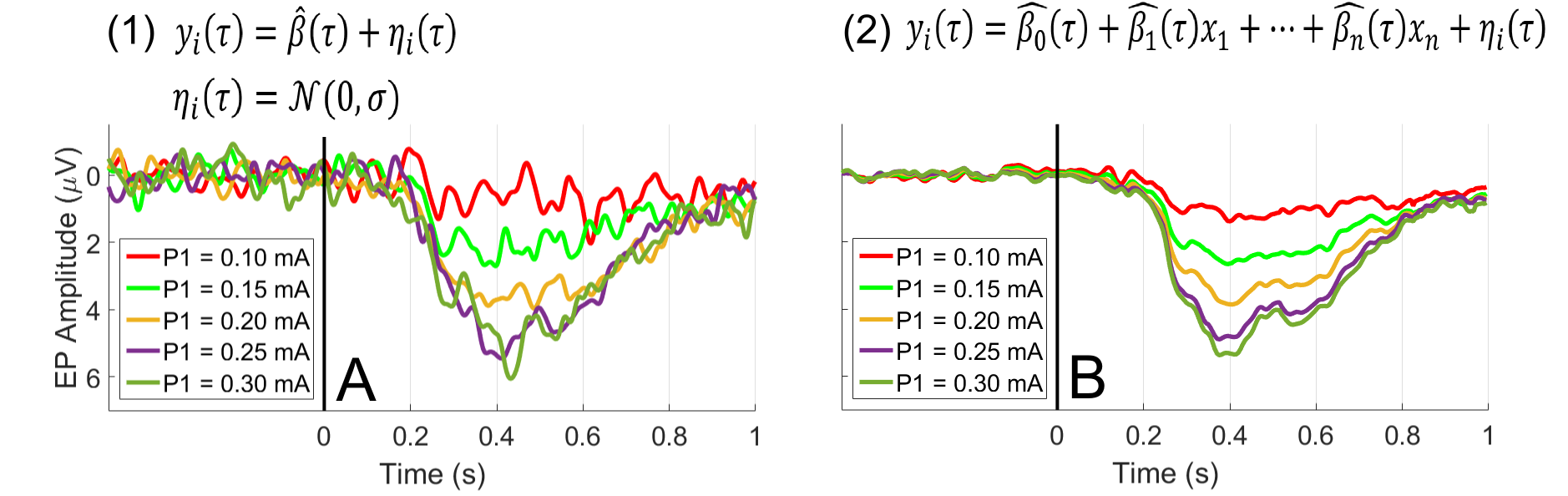
Figure 5
Future Research
Our first technologies are starting to find their way into clinical practice with the cooperation of companies and hospitals, and with the help of clinicians. However, there is still much work to be done. The current approach sheds light on variations in brain activity during perturbation of the pain system but is by no means as efficient and elegant as system identification approaches that are currently applied for the identification of electrical and mechanical systems. The problems encountered in developing such an approach are numerous:
-
To keep working on the development of state-of-the-art pain diagnostics we need the help of biomedical engineers, technical clinicians, electrical engineers and more. Our current roadmap includes several new challenges:
-
It turns out that for 72.5% of all stimuli, we can identify if somebody detected the stimulus based on the EEG signal by using a linear model. However, we can do better: we want to use machine learning techniques to develop an optimal brain-activity based estimator of stimulus detection.
-
We are one of the only groups in the world that apply system identification techniques on the human pain system. We would like to take our experiments to the next level by development of new system identification methods which efficiently use the data generated by stimulation and EEG recording.
-
The AmbuStim stimulator is not only used at the University of Twente, but also at several hospitals. Although the stimulator already successfully used for chronic pain research, we would like to continue improving the usability of the stimulator in the clinic and in research.
We have a wish-list including several improvements to stimulator hardware and software.
Are you interested in working on an assignment including technical and medical challenges? Do you want to work in the research group with the highest number of open access publications of the faculty EEMCS? And are you interested in working on one of these interesting topics? Please send an e-mail to j.r.buitenweg@utwente.nl or drop by at the lab.
References
-
Breivik, H., et al., Survey of chronic pain in Europe: Prevalence, impact on daily life, and treatment. European Journal of Pain, 2006. 10(4): p. 287-333.
-
Steenbergen, P., et al., Characterization of a bimodal electrocutaneous stimulation device, in 4th European Conference of the International Federation for Medical and Biological Engineering. 2008, EMBEC2008, Antwerp: Antwerp. p. 230-234.
-
Doll, R.J., et al., Effect of temporal stimulus properties on the nociceptive detection probability using intra-epidermal electrical stimulation. Experimental Brain Research, 2016. 234(1): p. 219-227.
-
Doll, R.J., et al., Responsiveness of electrical nociceptive detection thresholds to capsaicin (8 %)-induced changes in nociceptive processing. Experimental Brain Research, 2016. 234(9): p. 2505-2514.
-
Treede, R.D., Baumgartner, U., Monitoring Brain Electrical and Magnetic Activity for Assessing Pain: Advantages and Limitations, in The Brain Adapting with Pain, A.V. Apkarian, Editor. 2015.